Unlocking the Power of Video Annotation Tools for Machine Learning
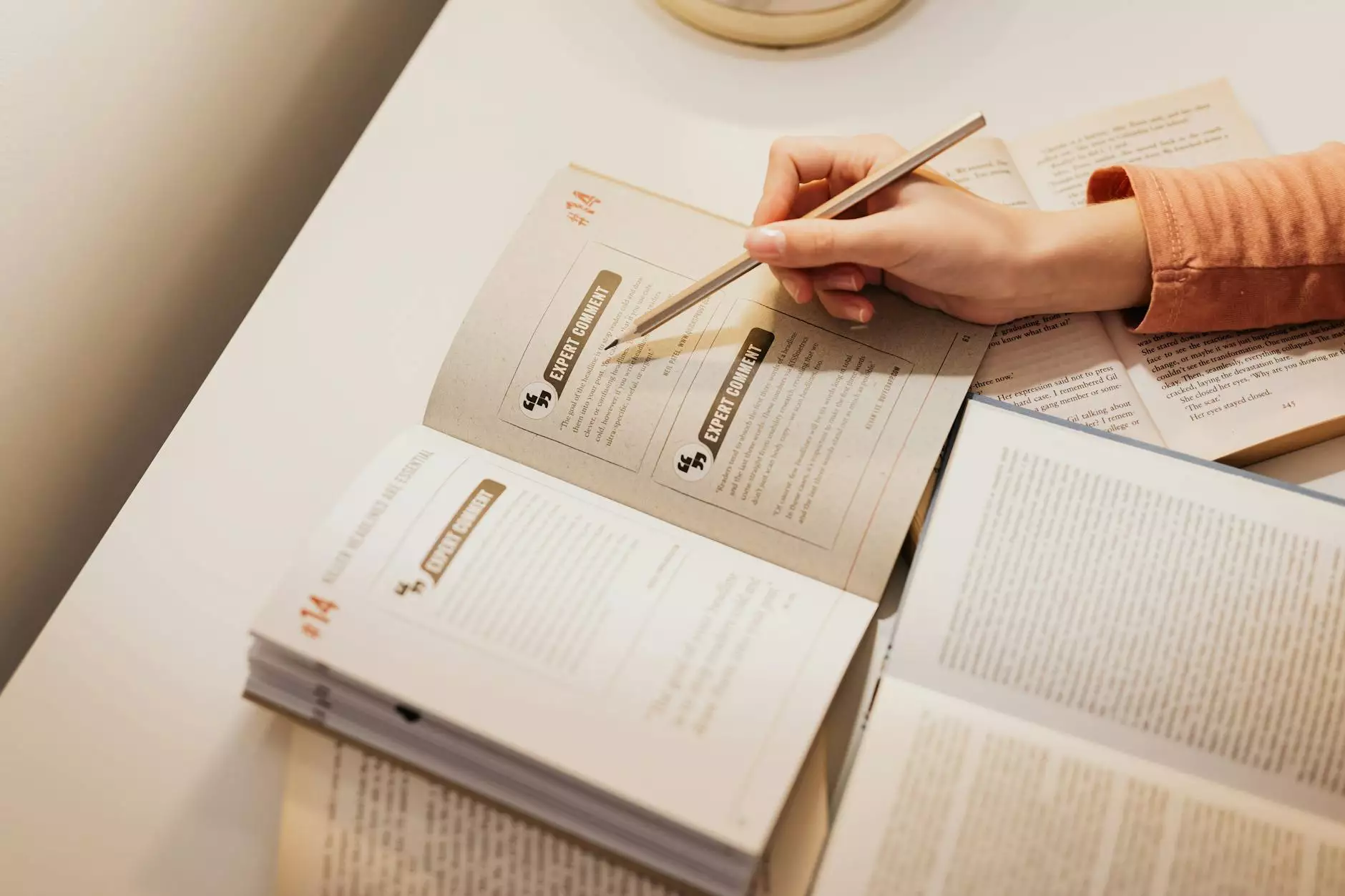
As we step further into the realm of artificial intelligence and machine learning, the importance of data annotation continues to crystallize. Among the myriad forms of data, video data holds a unique position, serving as a cornerstone for developing highly accurate machine learning models. Video annotation tools for machine learning emerge as vital resources in this landscape, empowering businesses to harness the potential of multimedia data effectively. In this article, we explore the significance of video annotation and how platforms like KeyLabs.AI stand out in this domain.
The Importance of Data Annotation in Machine Learning
Data annotation refers to the process of labeling or tagging data to make it understandable for machine learning models. This critical process forms the backbone of any AI application, as models learn to recognize patterns and make predictions based on the information fed to them. Here’s why data annotation is particularly relevant in the context of video data:
- Complexity of Video Data: Video contains a wealth of information—movement, interactions, and changes over time. Properly annotating this data allows machines to learn more nuanced behaviors.
- Increased Accuracy: Well-annotated datasets lead to more precise model predictions. Annotations help machines understand the context of visual information, enhancing their decision-making capabilities.
- Scalability: As businesses grow and data volumes increase, the need for scalable solutions like video annotation tools becomes paramount. Efficient annotation processes can handle large datasets without sacrificing quality or speed.
Types of Video Annotations
Video annotation tools employ various methods for tagging data, and understanding these types can help businesses choose the right approach for their needs:
1. Object Detection and Tracking
This involves identifying and tagging individual objects within video frames. For instance, in surveillance footage, tracking a person’s movement through different scenes can provide valuable insights for security and safety measures.
2. Action Recognition
Annotating actions helps models learn to recognize specific human activities (e.g., running, jumping). This is especially useful in sports analytics or health monitoring applications.
3. Event Detection
Event detection annotations focus on identifying noteworthy occurrences within a video. This could range from a specific interaction in social media clips to significant moments in live broadcasts.
4. Segmentation
Segmentation involves dividing video frames into meaningful segments, which can be classified or analyzed separately. This technique is often used in medical imaging or autonomous vehicle navigation.
Advantages of Using Video Annotation Tools for Machine Learning
Utilizing specialized video annotation tools brings numerous advantages that can significantly improve the efficiency and accuracy of your machine learning projects:
1. Streamlined Workflow
Video annotation tools automate many tedious tasks in the annotation process, allowing teams to focus on more strategic aspects of their projects.
2. Enhanced Collaboration
Many modern annotation platforms provide collaborative features, allowing multiple annotators to work simultaneously on projects. This facilitates faster project completion and a richer dataset.
3. Quality Control Features
To ensure high-quality annotations, leading tools incorporate quality control mechanisms. These features flag potential errors and allow for the review and correction of annotations.
4. Adaptability to Various Industries
Video annotation tools are versatile and can be adapted for diverse applications across industries, including healthcare, marketing, automotive, and sports.
Choosing the Right Video Annotation Tool
When searching for the best video annotation tool for machine learning, consider the following factors:
- User-Friendly Interface: An intuitive interface can save time and reduce the learning curve for new users.
- Customizability: Look for tools that allow for customizable workflows and annotation types to fit your specific project needs.
- Integration Capabilities: The tool should integrate easily with your existing data pipelines and machine learning frameworks.
- Support and Community: A strong user community and good customer support can enhance your experience and resolve issues quickly.
KeyLabs.AI: A Leading Video Annotation Platform
Among numerous video annotation solutions, KeyLabs.AI shines as a comprehensive data annotation platform dedicated to machine learning projects. Here’s what makes it a preferred choice:
1. Advanced Annotation Features
KeyLabs.AI offers an array of advanced annotation features tailored for video data. Users can easily perform object detection, action recognition, and event detection with precision.
2. AI-Assisted Annotation
The platform leverages AI algorithms to assist human annotators by providing smart suggestions, reducing the time spent on manual labeling while increasing accuracy.
3. Seamless Collaboration Tools
With KeyLabs.AI, teams can work together in real-time, ensuring quicker turnaround times for annotation projects. Each team member can access the platform from anywhere, promoting flexibility and agility.
4. Strong Focus on Security
Data security is crucial in today’s digital landscape. KeyLabs.AI implements robust security measures to protect user data and comply with industry regulations.
Real-World Applications of Video Annotation Tools
The transformative power of video annotation tools is evident across multiple sectors:
1. Healthcare
In healthcare, video annotation can be used for analyzing surgical procedures, identifying patient behaviors, and monitoring therapy sessions, leading to improved patient outcomes.
2. Automotive
For autonomous vehicles, video annotation is vital for training models to recognize road signs, pedestrians, and obstacles, thereby enhancing safety and navigation accuracy.
3. Retail
In retail environments, video footage can be analyzed to study customer behavior, optimize store layouts, and enhance marketing strategies based on interaction data.
4. Sports Analytics
Sports teams utilize video annotation to dissect gameplay, analyze player performance, and strategize for upcoming matches, allowing for refined training and optimization.
The Future of Video Annotation Tools
As artificial intelligence technologies evolve, the role of video annotation tools will expand significantly. The future will likely include:
1. Increased Automation
With advancements in machine learning, we can expect more automated annotation processes that require minimal human intervention, making data handling faster and more efficient.
2. Enhanced Interactivity
Future tools might incorporate virtual reality (VR) and augmented reality (AR) features, allowing annotators to engage with the data in innovative ways.
3. Improved Data Privacy Measures
As regulations around data usage tighten, video annotation tools will need to incorporate robust privacy features to ensure compliance while maintaining usability.
Conclusion
In conclusion, video annotation tools for machine learning represent a critical asset for businesses aiming to leverage multimedia data effectively. Platforms like KeyLabs.AI are leading the charge by providing comprehensive, user-friendly solutions that empower teams to annotate video data efficiently and accurately. As we look ahead, the evolution of these tools will undoubtedly introduce even greater capabilities, further enhancing the way we analyze and utilize video data for various applications.
Investing in advanced video annotation solutions is not just a choice; it is a strategic move towards unlocking the full potential of machine learning and AI. Embrace the future of data annotation with KeyLabs.AI today!